Robinhood CEO Vlad Tenev calls prediction markets 'the future' and says the company will play a 'leading role'
- today, 4:34 PM
- businessinsider.com
- 0
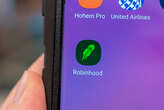
Since the launch of ChatGPT in late 2022 we’ve known that artificial intelligence language models are prone to spewing falsehoods, otherwise known as hallucinations.
The AI companies have been telling us that it’s a problem that can be cured. And with the use of technologies like retrieval-augmented generation (in which the AI calls on a database of reliable information), hallucinations have indeed decreased in many contexts. But they persist, as a recent Wired investigation of the Perplexity AI search tool illustrated. The Wired story features an especially bold headline: “Perplexity Is a Bullshit Machine.”
The use of “bullshit” made more than a grabby headline; it was a reference to recently published research from a trio of philosophy professors at Glasgow University. The report, titled “ChatGPT is bullshit,” argues that calling the false output of large language models hallucination is misleading; what LLMs are really spouting, they argue, is more like bullshit. And not just any bullshit: Bullshit as defined by the late moral philosopher Harry Frankfurt in his 2005 bestseller, On Bullshit.
Frankfurt’s book is principally concerned with defining the difference between a “liar” and a “bullshitter.” A bullshitter (aka “bullshit artist”) doesn’t use facts to come off as credible and therefore persuasive, he explained, but is content to say things that sound true to get the same result. For example, a used-car salesman who is practicing bullshit uses a set of talking points he thinks will lead someone to buy a car. Some of the talking points may be true, some may be false; to him it doesn’t matter: He would use the same set of points whether they happened to be true or not. “[He] does not reject the authority of the truth, as the liar does, and oppose himself to it,” Frankfurt wrote. “He pays no attention to it at all. By virtue of this, bullshit is a greater enemy of the truth than lies are.”
In their recent report, the Glasgow researchers—Michael Townsen Hicks, James Humphries, and Joe Slater—argue that Frankfurt’s bullshit definition fits the behavior of LLMs better than the term hallucinate. In order to hallucinate, the researchers argue, one must have some awareness or regard for the truth; LLMs, by contrast, work with probabilities, not binary correct/incorrect judgments. Based on a huge many-dimensional map of words created by processing huge amounts of text, LLMs decide which words (based on meaning and current context) would most likely follow from the words used in a prompt. They’re inherently more concerned with sounding truthy than delivering a factually correct response, the researchers conclude.
Importantly, the LLM doesn’t always choose the word that is statistically most likely to follow. Letting the model choose between a set of more or less likely candidates for the next word gives the output an unexpected, creative, or even human quality. This quality can even be modulated using a control that AI developers call “temperature.” But dialing up the model’s temperature increases the chances it will generate falsehoods.
When LLMs “hallucinate,” it’s normally because they’ve encountered a dearth of information about the particular subject or context within their training data. They rely on their existing probability settings and, in a sense, wing it. Frankfurt’s “bullshit” definition seems to capture this behavior remarkably well. “Bullshit is unavoidable whenever circumstances require someone to talk without knowing what he is talking about,” he wrote.
And these metaphors matter, the researchers say. “Calling their mistakes ‘hallucinations’ isn’t harmless: It lends itself to the confusion that the machines are in some way misperceiving but are nonetheless trying to convey something that they believe or have perceived,” they write, concluding that the term also “feeds into overblown hype about their abilities among technology cheerleaders.”
Of course, Frankfurt was talking about human bullshitters, not AIs. But human metaphors are the best tools we have for understanding AI. Because of the opaque and/or alien quality of the inner workings of LLMs, the public has relied on AI researchers and Big Tech companies to provide those metaphors. That might have been fine before generative AI became a very big business—and before there was a profit motive to spin and control narratives. With profit-hungry companies like Google and OpenAI choosing their words carefully, AI regulators and consumers alike should insist on hearing a diversity of opinions, especially when it involves crucial issues such as bias and safety.
No comments